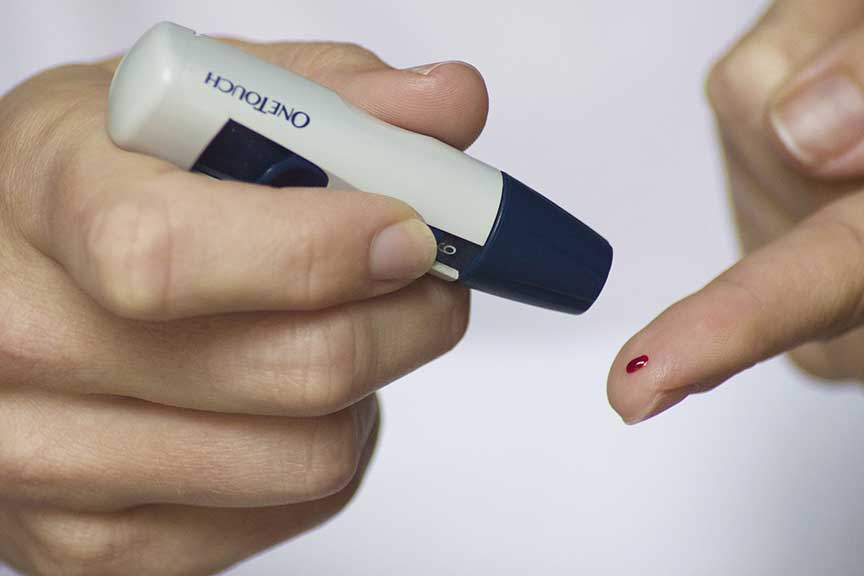
Medial EarlySign (earlysign.com), a leader in machine-learning based solutions to aid in early detection and prevention of high-burden diseases, announced its first suite of diabetes risk predictors for healthcare organizations. Expanding the company’s portfolio of clinical risk predictors, these new diabetes-focused AlgoMarkers are designed to help healthcare systems identify and engage patients at high risk for diabetes and downstream complications.
The initial suite includes EarlySign’s Pre2D AlgoMarker™ solution to identify pre-diabetic patients at highest risk of progressing to diabetes within a one-year period; and the Diabetes to CKD AlgoMarker™, which identifies type 2 diabetic patients at high risk for developing stage 2-4 chronic kidney disease (CKD) within three years.
“In the U.S. alone, approximately 1.5 million pre-diabetic adults will become diabetic this year, while between 20% and 40% of diabetic patients worldwide suffer from diabetes-related kidney complications,” said Ori Geva, CEO of Medial Early Sign. “Our Pre2D™ and Diabetes to CKD™ solutions provide healthcare systems opportunities to identify and reach out to high-risk patients within an actionable timeframe, when preventative measures can be initiated, and resources allocated to potentially delay or prevent the onset of disease.”
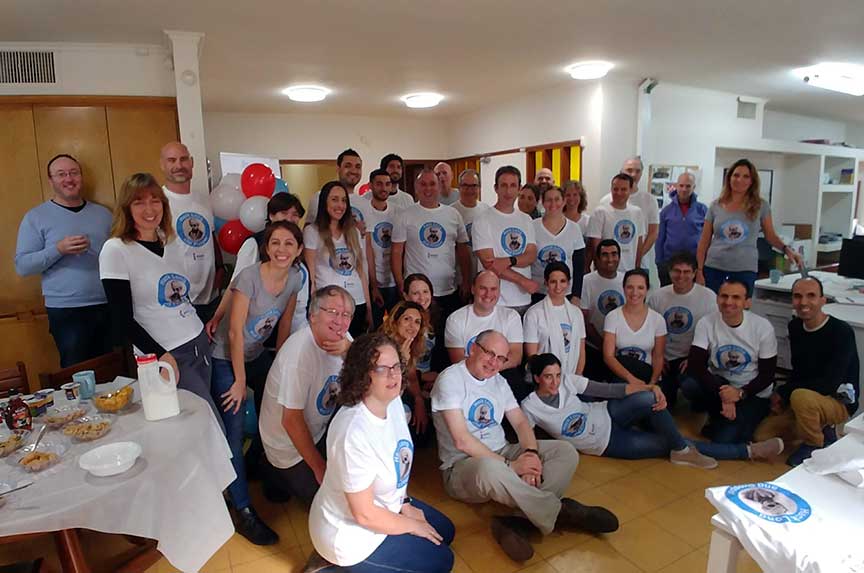
EarlySign’s Pre2D™ predictive solution applies advanced machine learning-based algorithms to identify “hidden signals” residing in existing, routine blood tests. Factoring in age, gender and BMI – and requiring no special patient preparation — it flags those pre-diabetic patients at high risk for progressing to diabetes in one (1) year or less. In a retrospective data study of 1.1 million pre-diabetic patients, the Pre2D AlgoMarker flagged the top 10% of the pre-diabetic population at risk and successfully identified 58.3% of patients who became diabetic within a 12-month period. This is a 14.7% increase over a logistic regression model that, by flagging 10% of the population, identified only 43.6% of future diabetics.
The Diabetes to CKD™ risk predictor uses basic demographic data, routine lab results, diagnostic codes, and medication information to flag type 2 diabetic patients most likely to develop stages 2-4 of chronic kidney disease in 3 years or less. In a retrospective data study of hundreds of thousands of diabetic patients, the algorithm was able to capture 25.5% of those most likely to progress to CKD within three years, by flagging only 3% of the diabetic population. This amounts to 77% more patients than would have been identified if the last eGFR value was used.